ליבנת ג'רבי ארנון 2013-2014
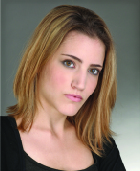
- מוסד לימודים לדוקטורט:
- אוניברסיטת תל-אביב
- תחום אקדמי:
- ביואינפורמטיקה
- מנחה/מנחים בדוקטורט:
- פרופ' איתן רופין
- נושא הדוקטורט:
- Genome-scale Modelling of Cancer Genetics and Metabolism Towards the Identification of Selective Anticancer Treatments
- מוסד בתר-דוקטורט:
- מכון ברוד
- מוסד נוכחי:
- מכון ברוד
- משרה אקדמית נוכחית:
- בתר-דוקטורנטית
- כתובת דוא"ל:
- livnat.jerby@gmail.com
- קורות חיים
- פרסומים
- עמוד הבית
Intrigued by the mysteries of the human body, Livnat started her academic studies at the Faculty of Life Sciences. During the second year of her studies she became increasingly more aware of the technological advancements that revolutionized the conservative biological research, and hence turned to study also computer science.
Since Livnat finished her BSc in Life and Computer Science at Tel-Aviv University, she has been working under the supervision of Prof. Eytan Ruppin, in collaboration with experimental groups, worldwide, to systematically study the unique genetic and metabolic characteristics of cancer.
Her research agenda is primarily focused on basic scientific questions, but her main goal is to help provide a basis for rational drug discovery. In her current PhD research, Livnat performed the first genome-scale study of breast cancer metabolic progression by profiling the traits of individual patients' tumors. These profiles enabled her to explore underlying mechanisms of the disease, classify patients according to their prognosis and identify potential metabolic biomarkers. The latter are non-invasive, cost-effective means for early diagnosis and monitoring treatment efficacy.
More recently, Livnat has been working on a new approach to identify the unique vulnerabilities of cancer cells based on their genetic profiles. The basic idea is to exploit the genetic instability of the cancer to selectively target it without harming healthy cells. To this end Livnat has been developing a framework to map the interplay between different genes in cancer on a genome-scale. The latter enables to translate between genomic data and therapeutic outcomes in cancer and could help developing more effective treatment strategies with fewer side-effects.